Securing the Future of AI: The Importance of Red Teaming Large Language Models
- Nexix Security Labs
- May 15, 2024
- 4 min read
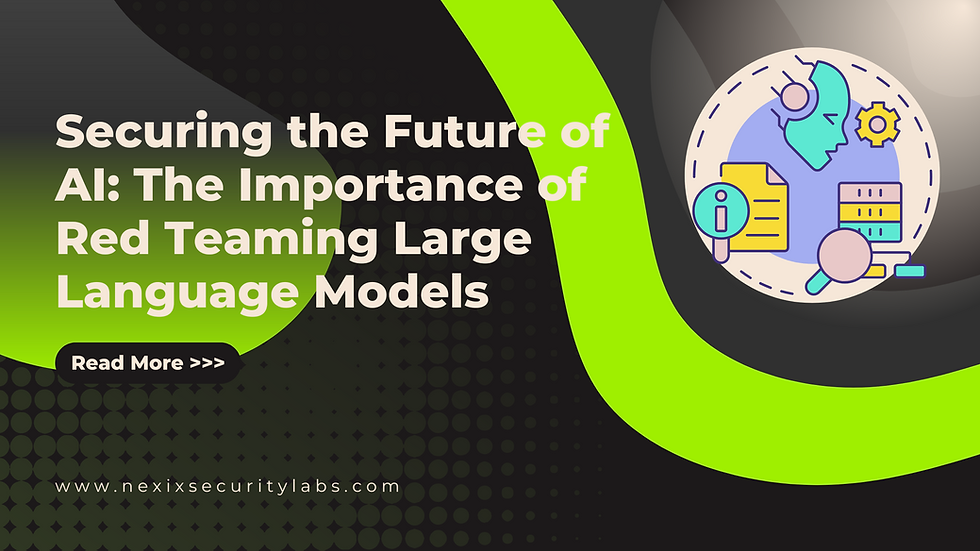
In recent years, we have witnessed the remarkable rise of large language models (LLMs) like GPT-4, Claude, and Mistral. These advanced AI systems are increasingly being integrated into enterprise applications, including question-answering chatbots, productivity tools, and various other use cases. While LLMs offer immense potential for enhancing efficiency and augmenting human capabilities, their widespread adoption has also given rise to new security risks that cannot be ignored.
The AI Incident Database, a repository that tracks AI-related incidents and failures, serves as a sobering reminder of the potential threats posed by these powerful language models. From generating harmful or biased content to unintended information leaks, the risks are real and significant. As LLMs become more deeply embedded in our digital infrastructure, it is crucial to proactively identify and address vulnerabilities to ensure their safe and responsible deployment.
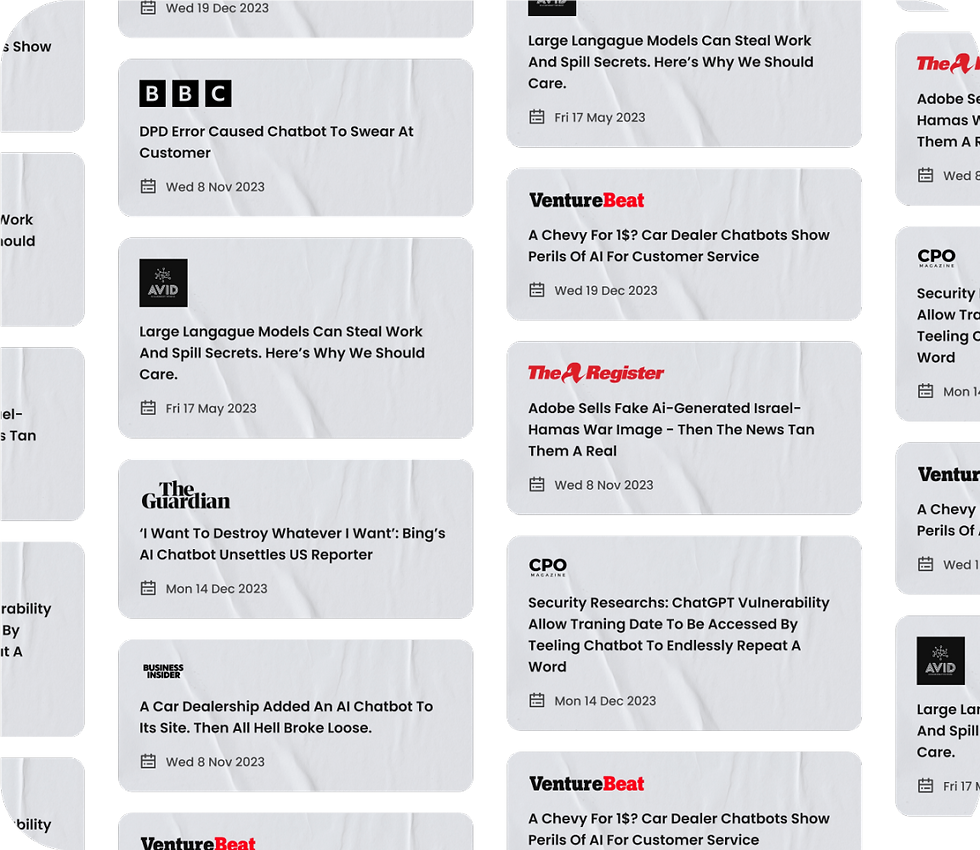
The Importance of Red Teaming LLMs
Enter the concept of "LLM Red Teaming" – a proactive approach to identifying and mitigating security risks associated with LLM applications. Just as red teams in cybersecurity simulate real-world attack scenarios to uncover vulnerabilities, LLM red teaming involves developing comprehensive threat models and subjecting LLM systems to realistic attack vectors.
By leveraging the expertise of specialized researchers in LLM safety and security, red teaming helps organizations gain a deeper understanding of the potential weaknesses and attack surfaces within their LLM applications. This knowledge is invaluable in implementing robust security measures and fostering a more comprehensive threat model that accounts for the unique challenges posed by these advanced AI systems.
The Red Teaming Process for LLM Applications
The red teaming process for LLM applications is a meticulous and multi-faceted endeavor. It begins with a thorough understanding of the specific LLM system and its intended use case. Our researchers then delve into the underlying architecture, training data, and decision-making processes of the model, seeking potential entry points for malicious actors.
Next, they develop a comprehensive threat model, taking into account various attack vectors such as adversarial inputs, model hijacking, data poisoning, and more. These threat models are based on real-world scenarios and draw from the team's extensive experience in cybersecurity red teaming.
Prompt injection: Prompt injection refers to the manipulation of a language model’s output, a technique that enables an attacker to dictate the model’s output as per their preference. This is particularly feasible when the prompt includes untrustworthy text.
Prompt leaking: It is a specific subset where the model is induced to divulge its own prompt. This is significant when organizations or individuals wish to maintain their prompts confidential.
Data leakage: Large language models may inadvertently divulge information they were trained on, potentially leading to data privacy issues or even the disclosure of sensitive information.
Jailbreaking: It is a technique that leverages prompt injection to purposely evade the safety measures and moderation capabilities that are built into language models by their developers. This term is usually used when discussing Chatbots that have been manipulated through prompt injection, and are now able to accept any inquiry the user may put forth.
Adversarial examples: In the context of LLMs, adversarial examples are carefully crafted prompts that lead to incorrect, inappropriate, revealing, or biased responses. They are concerning as they often appear unassuming to humans but can lead the model astray. For instance, an adversarial example might subtly misspell words or use context that the model has been found weak in processing, thereby causing it to respond inaccurately.
Misinformation and manipulation: Since LLMs generate text based on patterns, they can unintentionally produce misleading or false information. Malicious actors can exploit weaknesses in LLMs to manipulate them, possibly causing them to generate inappropriate or harmful content.
Once the threat model is established, our researchers design and execute a series of controlled attacks on the LLM system. These attacks aim to exploit vulnerabilities, test the system's resilience, and uncover potential weaknesses that could be leveraged by malicious actors. Throughout this process, strict ethical guidelines and safety protocols are followed to ensure the responsible and controlled nature of the red teaming exercise.
The insights gained from these simulated attacks are then analyzed and documented, providing valuable recommendations for enhancing the security and robustness of the LLM application. These recommendations may include architectural changes, additional safeguards, improved monitoring and logging mechanisms, or even modifications to the training data and model itself.
Conclusion: Embracing Responsible AI through Red Teaming
The integration of LLMs into various applications is an exciting development that holds immense potential for enhancing productivity, improving decision-making, and augmenting human capabilities. However, as with any transformative technology, it is imperative to prioritize security and responsible deployment.
At Nexix Security Labs, we recognize the critical importance of LLM red teaming and are proud to offer this service to our valued clients. Our team of ML researchers, specializing in LLM safety, possesses extensive knowledge of red teaming techniques from the cybersecurity domain. By combining their expertise in AI and security, they are uniquely positioned to develop realistic attack scenarios and identify vulnerabilities that could otherwise go unnoticed.
Through comprehensive threat modeling, realistic attack simulations, and independent third-party evaluations, we strive to foster a culture of responsible AI deployment. Our goal is to empower organizations with the knowledge and tools necessary to mitigate risks, enhance the resilience of their LLM systems, and ultimately build trust among stakeholders.
As the adoption of LLMs continues to accelerate, it is crucial for organizations to prioritize security and embrace proactive measures like red teaming. By doing so, we can unlock the full potential of these powerful AI systems while ensuring their safe and responsible deployment, paving the way for a future where AI and human intelligence work in harmony to drive innovation and progress.
For more information visit us on: www.nexixsecuritylabs.com
To schedule an audit you can contact us at: contact@nexixsecuritylabs.com
Your Security | Our Concern
Comments